Is it just me, or has ChatGPT-4 taken a nosedive when it comes to legal research and writing? There has been a noticeable decline in its ability to locate primary authority on a topic, analyze a fact pattern, and apply law to facts to answer legal questions. Recently, instructions slide through its digital grasp like water through a sieve, and its memory? I would compare it to a goldfish, but I don’t want to insult them. And before you think it’s just me, it’s not just me, the internet agrees!
ChatGPT’s Sad Decline
One of the hottest topics in the OpenAI community, in the aptly named GPT-4 is getting worse and worse every single update thread, is the perceived decline in the quality and performance of the GPT-4 model, especially after the November 2023 update. Many users have reported that the model is deteriorating with each update, producing nonsensical, irrelevant, or incomplete outputs, forgetting the context, and ignoring instructions. Some users have even reverted to previous versions of the model or cancelled their subscriptions. Here are some specific quotations from recent comments about the memory problem:
- December 2023 – “I don’t know what on Earth is wrong with GPT 4 lately. It feels like I’m talking to early 3.5! It’s incapable of following basic instructions and forgets the format it’s working on after just a few posts.”
- December 2023 – “It ignores my instructions, in the same message. I can’t be more specific with what I need. I’m needing to repeat how I’d like it to respond every single message because it forgets, and ignores.”
- December 2023 – “ChatGPT-4 seems to have trouble following instructions and prompts consistently. It often goes off-topic or fails to understand the context of the conversation, making it challenging to get the desired responses.”
- January 2024 – “…its memory is bad, it tells you search the net, bing search still sucks, why would teams use this product over a ChatGPT Pre Nov 2023.”
- February 2024 – “It has been AWFUL this year…by the time you get it to do what you want format wise it literally forgets all the important context LOL — I hope they fix this ASAP…”
- February 2024 – “Chatgpt was awesome last year, but now it’s absolutely dumb, it forgets your conversation after three messages.”
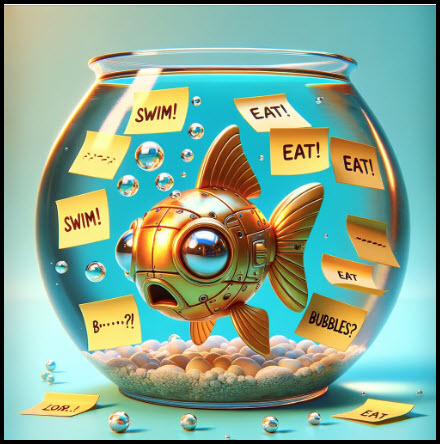
OpenAI has acknowledged the issue and released an updated GPT-4 Turbo preview model, which is supposed to reduce the cases of “laziness” and complete tasks more thoroughly. However, the feedback from users is still mixed, and some are skeptical about the effectiveness of the fix.
An Example of Confusion and Forgetfulness from Yesterday
Here is one of many examples of my experiences which provide an illustrative example of the short-term memory and instruction following issues that other ChatGPT-4 users have reported. Yesterday, I asked it to find some Texas cases about the shopkeeper’s defense to false imprisonment. Initially, ChatGPT-4 retrieved and summarized some relatively decent cases. Well, to be honest, it retrieved 2 relevant cases, with one of the two dating back to 1947… But anyway, the decline in case law research ability is a subject for another blog post.
Anyway, in an attempt to get ChatGPT-4 to find the cases on the internet so it could properly summarize them, I provided some instructions and specified the format I wanted for my answers. Click here for the transcript (only available to ChatGPT-4 subscribers).
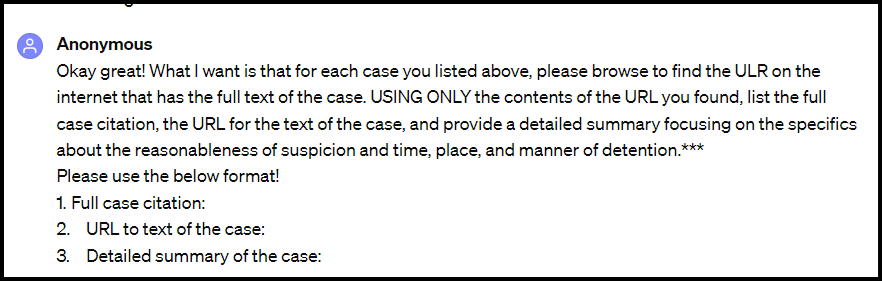
Confusion ran amok! ChatGPT-4 apparently understood the instructions (which was a positive sign) and presented three cases in the correct format. However, they weren’t the three cases ChatGPT had listed; instead, they were entirely irrelevant to the topic—just random criminal cases.
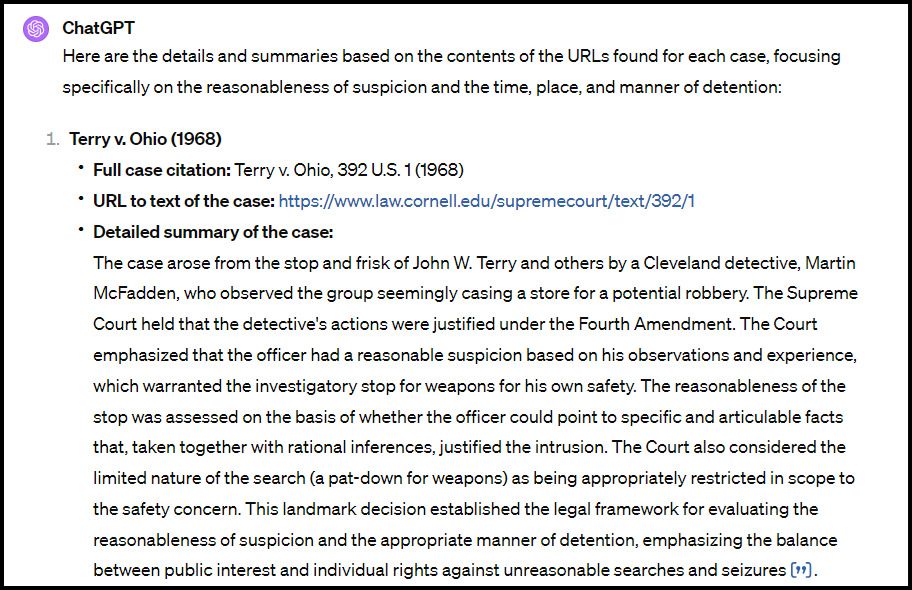
It remembered… and then forgot. When reminded that I wanted it to work with the first case listed and provided the citation, it apologized for the confusion. It then proceeded to give the correct citation, URL, and a detailed summary, but unfortunately in the wrong format!
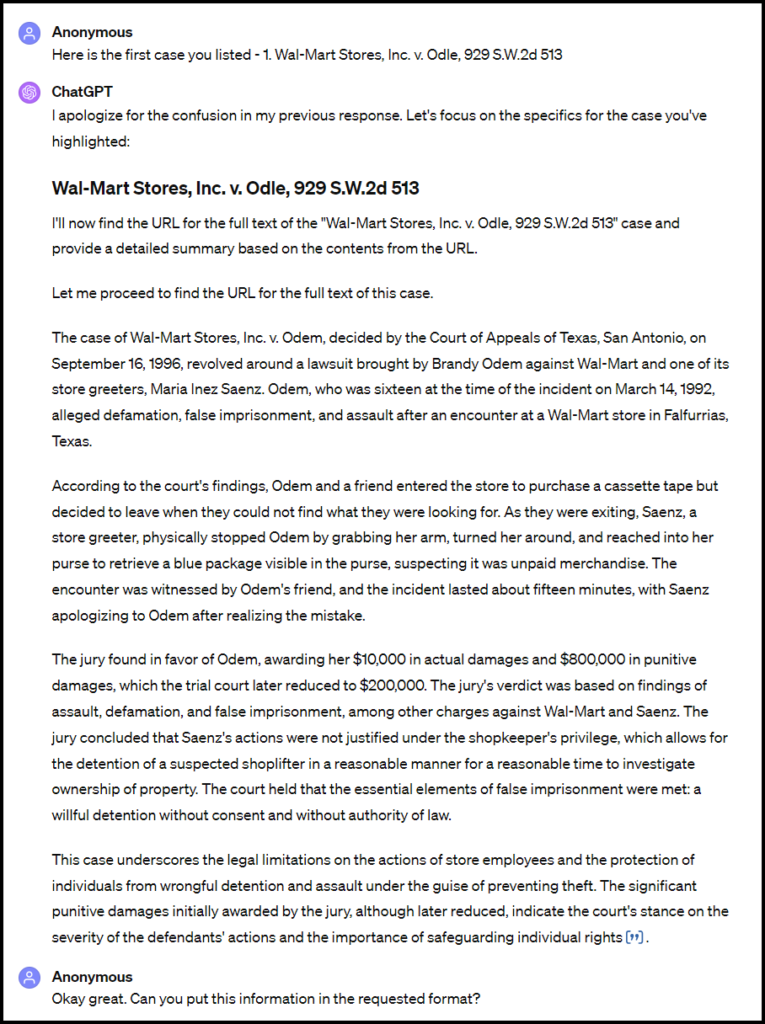
Eventually, in a subsequent chat, I successfully got it to take a case it found, locate the text of the case on the internet, and then provide the information in a specified format. However, it could only do it once before completely forgetting about the specified format. I had to keep cutting and pasting the instructions for each subsequent case.
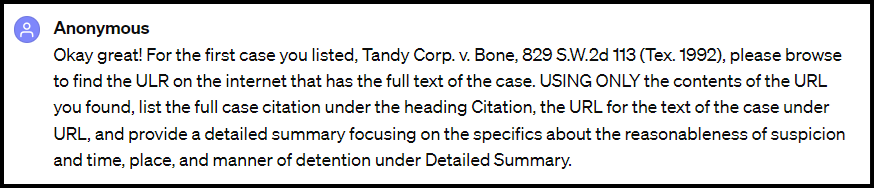
Sigh… I definitely echo the sentiments of expressed on the GPT-4 is getting worse and worse every single update thread.
ChatGPT Is Growing a Long Term Memory
Well, the news is not all bad! While we are on the topic of memory, OpenAI has introduced a new feature for ChatGPT – the ability to remember stuff over time. ChatGPT’s memory feature is being rolled out to a small portion of free and Plus users, with broader availability planned soon. According to OpenAI, this enhancement allows ChatGPT to remember information from past interactions, resulting in more personalized and coherent conversations. During conversations, ChatGPT automatically picks up on details it deems relevant to remember. Users can also explicitly instruct ChatGPT to remember specific information, such as meeting note preferences or personal details. Over time, ChatGPT’s memory improves as users engage with it more frequently. This memory feature could be useful for users who want consistent responses, such as replying to emails in a specific format.
The memory feature can be turned off entirely if desired, giving users control over their experience. Deleting a chat doesn’t erase ChatGPT’s memories; users must delete specific memories individually…which seems a bit strange – see below. For conversations without memory, users can use temporary chat, which won’t appear in history, won’t use memory, and won’t train the AI model.
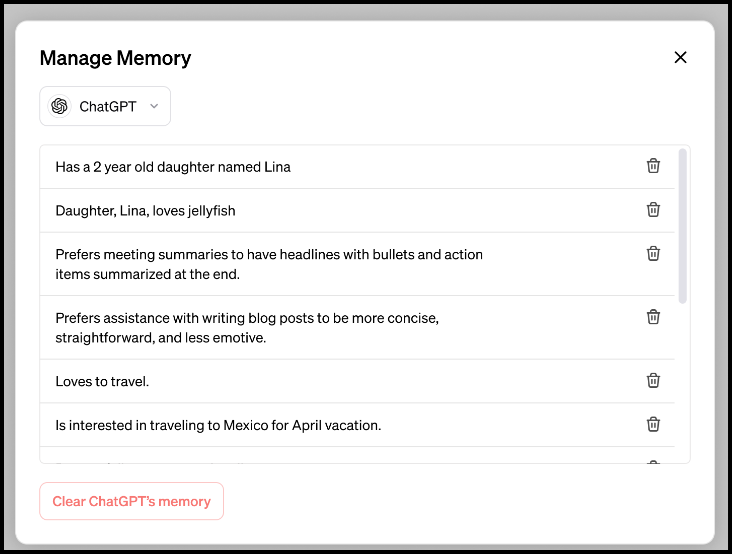
The Future?
As we await improvements to our once-loved ChatGPT-4, our options remain limited, pushing us to consider alternative avenues. Sadly, I’ve encountered recent similar shortcomings with the once-useful for legal research and writing Claude 2. In my pursuit of alternatives, platforms like Gemini, Perplexity, and Hugging Face have proven less than ideal for research and writing tasks. However, amidst these challenges, Microsoft Copilot has shown promise. While not without its flaws, it recently demonstrated adequate performance in legal research and even took a passable stab at a draft of a memo. Given OpenAI’s recent advancements in the form of Sora, the near-magical text-to-video generator that is causing such hysteria in Hollywood, there’s reason to hope that they can pull ChatGPT back from the brink.